Reflections on AI Explain A Postmortem
In this exploration, we delve into the aftermath of AI projects, analyzing what went wrong and how to learn from those experiences. By examining postmortem reports, we gain critical insights into the successes and failures of artificial intelligence initiatives. This reflection offers a balanced view of the challenges AI systems face, from ethical concerns to technical breakdowns, and how they can be addressed in future developments.
The rapid advancement of artificial intelligence has led to significant breakthroughs across various sectors, transforming the way we interact with technology and one another. Among these innovations, AI Explain stands out as a project that aimed to enhance the interpretability and transparency of AI systems. However, as with many ambitious undertakings, AI Explain encountered challenges that ultimately led to its decline. This postmortem explores the lessons learned, the hurdles faced, and the potential paths forward in the realm of AI explainability.
Understanding AI Explain
AI Explain was designed to demystify the often opaque nature of AI decision-making processes. With the increasing reliance on AI systems, the demand for transparency grew. Stakeholders, including businesses, developers, and users, expressed the need to understand how AI models arrived at specific conclusions. The core objective of AI Explain was to provide insights into the inner workings of AI algorithms, fostering trust and accountability in their outputs.
The Importance of Explainability in AI
Explainability is critical in building trust between humans and AI systems. Users must feel confident that AI-driven decisions are fair, unbiased, and based on sound reasoning. Without explainability, the potential for misuse and misunderstanding increases, leading to negative societal impacts. For example, in sensitive areas like healthcare, finance, and law enforcement, a lack of transparency can result in biased outcomes or reinforce existing inequalities.
Initial Goals and Vision
The vision behind AI Explain was ambitious. The project aimed to create a comprehensive framework that would enable developers and organizations to interpret AI models effectively. The initial goals included developing user-friendly tools, creating educational resources, and fostering a community of practice around AI explainability. By making AI systems more interpretable, AI Explain sought to empower users to make informed decisions based on AI outputs.
Challenges Faced by AI Explain
Despite its noble intentions, AI Explain encountered several significant challenges that hindered its progress and eventual sustainability. These challenges can be categorized into technical, organizational, and societal dimensions.
Technical Challenges
One of the primary technical challenges faced by AI Explain was the complexity of AI models themselves. As AI algorithms, particularly deep learning models, became more intricate, explaining their decision-making processes became increasingly difficult. While simpler models like linear regression are relatively easy to interpret, the black-box nature of deep learning poses significant hurdles.
Additionally, the tools and frameworks available for explainability were often not mature enough to meet the project’s needs. The landscape of AI explainability is constantly evolving, and AI Explain struggled to keep pace with the rapid advancements in AI research and technology.
Organizational Challenges
On an organizational level, AI Explain faced difficulties in aligning stakeholders with varying interests. While some organizations recognized the importance of explainability, others prioritized performance metrics over transparency. This divergence in priorities created a lack of consensus around the project's goals, leading to fragmentation and inefficiencies.
Moreover, securing buy-in from decision-makers was an ongoing struggle. Many organizations hesitated to invest in explainability initiatives, viewing them as supplementary rather than essential. This reluctance to prioritize explainability ultimately stunted the growth of AI Explain.
Societal Challenges
Societal challenges also played a role in the decline of AI Explain. The public’s perception of AI is often colored by sensationalized narratives and fears surrounding job displacement and privacy concerns. This skepticism made it difficult for AI Explain to gain traction, as stakeholders questioned the efficacy and intentions of AI systems.
Furthermore, the disparity in access to AI technology and resources exacerbated the challenges faced by AI Explain. Smaller organizations and underserved communities often lacked the knowledge and tools necessary to engage with AI effectively. This digital divide highlighted the need for inclusive approaches to explainability, ensuring that all stakeholders could benefit from AI advancements.
Lessons Learned from AI Explain
Despite its eventual decline, the AI Explain initiative provided valuable insights into the broader landscape of AI explainability. Reflecting on its journey reveals several key lessons that can inform future efforts in the field.
The Necessity of Collaboration
One of the most significant takeaways from the AI Explain experience is the importance of collaboration across various sectors. Fostering partnerships between academia, industry, and policymakers can drive innovation in explainability efforts. Collaborative initiatives can lead to the development of shared resources, best practices, and standards that enhance transparency in AI systems.
Emphasizing User-Centric Design
AI Explain highlighted the need for user-centric design in developing explainability tools. It is essential to prioritize the needs and perspectives of end-users, ensuring that tools are intuitive and accessible. Engaging with diverse user groups can provide valuable insights into their requirements, ultimately leading to more effective explainability solutions.
Balancing Performance and Transparency
Striking a balance between performance and transparency is critical. While high-performing AI models are essential, organizations must also recognize the importance of explainability. The AI Explain experience underscores the need for a cultural shift in which transparency is viewed as a fundamental aspect of AI development rather than an afterthought.
Fostering Public Trust
Building public trust in AI systems is paramount for their successful integration into society. AI Explain emphasized the necessity of clear communication around AI decision-making processes and the potential implications of AI outputs. Engaging in open dialogues with the public can help demystify AI technologies and alleviate concerns.
Addressing Ethical Considerations
The ethical implications of AI decision-making cannot be overlooked. AI Explain's decline underscored the importance of integrating ethical considerations into the development and deployment of AI systems. Organizations must prioritize fairness, accountability, and transparency in their AI initiatives, ensuring that they do not perpetuate biases or exacerbate existing inequalities.
Potential Paths Forward
While the decline of AI Explain is a poignant reminder of the challenges associated with AI explainability, it also opens up opportunities for future endeavors in this field. Building on the lessons learned, several potential paths forward can be envisioned.
Developing Standardized Frameworks
The establishment of standardized frameworks for AI explainability can provide a roadmap for organizations seeking to enhance transparency. These frameworks should outline best practices, guidelines, and metrics for evaluating the explainability of AI systems. By creating a common language around explainability, stakeholders can work together more effectively.
Investing in Research and Development
Investing in research and development is crucial for advancing AI explainability. As the field continues to evolve, ongoing research can lead to the creation of more sophisticated tools and methodologies for interpreting AI models. Collaboration between academia and industry can foster innovation and drive progress in this area.
Promoting Education and Awareness
Raising awareness and educating stakeholders about the importance of AI explainability is essential for fostering a culture of transparency. Educational initiatives can empower individuals and organizations to engage meaningfully with AI systems, promoting informed decision-making and responsible usage.
Engaging Diverse Stakeholders
Engaging diverse stakeholders in discussions around AI explainability can lead to more inclusive solutions. By incorporating perspectives from various sectors and communities, organizations can ensure that their explainability efforts address the needs and concerns of all users.
The journey of AI Explain serves as a reflection on the complexities of AI explainability. While the initiative faced numerous challenges that ultimately led to its decline, the lessons learned are invaluable. Emphasizing collaboration, user-centric design, and ethical considerations can guide future efforts in enhancing the transparency of AI systems.
As we continue to navigate the evolving landscape of artificial intelligence, the importance of explainability remains paramount. By prioritizing transparency and fostering a culture of trust, we can harness the potential of AI for the betterment of society. The reflections on AI Explain are not merely a postmortem but a call to action for all stakeholders to work together towards a future where AI is both powerful and accountable.
Get in Touch
Website – https://www.webinfomatrix.com
Mobile - +91 9212306116
Whatsapp – https://call.whatsapp.com/voice/9rqVJyqSNMhpdFkKPZGYKj
Skype – shalabh.mishra
Telegram – shalabhmishra
Email - info@webinfomatrix.com
What's Your Reaction?
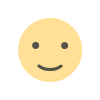
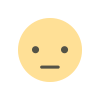
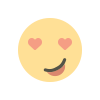
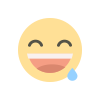
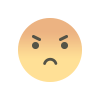
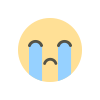
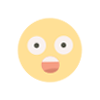